Media Query Source: Part 33 - TechTarget (US digital magazine); Hadoop vs Spark: An an-depth big data framework comparison
- TechTarget (US digital magazine)
- Apache Hadoop & Apache Spark
- Overlap can exist between installations
- Performance & use cases
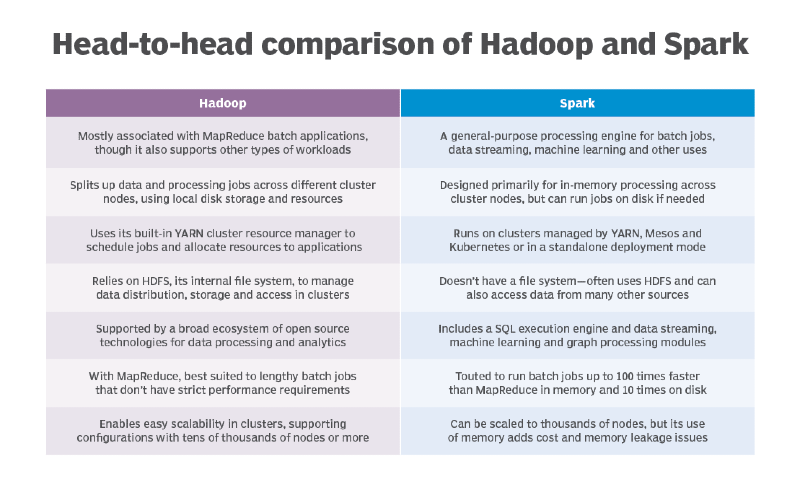
My responses ended up being included in an article at TechTarget (May 7, 2021). Extent of verbatim quote highlighted in orange, paraphrased quote highlighted in gray. Above image from cited article.
The responses I provided to a media outlet on March 15, 2021:
From a high level perspective, Spark can also process vast amounts of data by splitting it up and processing across distributed cluster nodes, but it typically does so much more quickly because its processing is performed in memory. And because Spark processes data in memory, it can satisfy use cases that Hadoop cannot, such as data streaming. Somewhat confusing is a component called Hadoop Streaming, which does not provide data streaming, but instead provides the ability to write MapReduce jobs in languages other than Java. Spark also supports multiple languages, but in a consistent manner that Hadoop does not provide, using APIs which resolve to common underlying code.
Applications and use cases: While the use cases to use Hadoop and Spark overlap for batch, this isn’t the case for others. For example, Spark enables streaming of data for use cases in which decisions need to be made quickly. Some refer to this type of processing as “real-time”, but since there are so many interpretations as to what this means I typically don’t use this term, instead referring to this type of processing as non-scheduled and non-batch, subsequently exploring what is really needed in terms of timing. While other frameworks also offer the ability to stream data, some faster than Spark, Spark enables doing so for other use cases using a common framework.